As part of Airbus’s ongoing efforts to advance automation in commercial aviation, the aircraft manufacturer’s Acubed innovation unit in Silicon Valley has now collected data on more than 100 airports across the U.S. The massive data collection campaign, which began in California in 2019 and expanded to the eastern U.S. last year, will help to enable autonomous flight through artificial intelligence (AI) and machine learning, according to the Europe-based aerospace group.
Over four months, Acubed flew its so-called flight test lab aircraft—a Beechcraft Baron 58 modified with cameras and sensors—to more than 100 airports across the country in various weather and lighting conditions. At each airport, the experimental aircraft collected copious amounts of imagery that the Acubed team is using to train and test machine-learning algorithms.
Using the real-world data collected during the campaign, the Acubed team can also generate synthetic datasets to create a more robust data pool for the machine-learning algorithms to assimilate. “To go collect the data to the level that we need for each airport would be impossible,” said Paul Smith, Acubed’s director of flight test operations. By combining synthetic data with real-world data, researchers can simulate a wider variety of conditions and scenarios, such as obstacles on a runway.
“We have two challenges,” Smith said. “One is to build the synthetic data and the other is to prove that the synthetic data we build will replicate real life in the models we are running. So what we’re doing is taking the real data that we collect in the airplane and comparing it to the synthetic data in enough cases for a regulator to find acceptable.”
To decide which airports to include in the data collection campaign, the Acubed team looked at airports in the U.S. where the Airbus A320 airliner operates. The A320 is the highest-selling single-aisle airliner, with more than 10,000 aircraft currently in service around the world. Airbus intends to give airliners such as the A320 family the capability to taxi, take off, and land autonomously, thereby reducing pilot workload while improving operational efficiency and safety.
“One hundred airports symbolize so much more than meets the eye. It stands for over 5 million images that will be funneled through our machine learning development pipeline to develop and test the robustness of our algorithms,” said Cedric Cocaud, chief engineer of Acubed’s Wayfinder group, which is overseeing the data project.
In addition to autonomous flight control systems, Airbus is applying its AI research to air traffic control and ground operations at airports. In September Acubed entered into a memorandum of understanding with Dallas Fort Worth International Airport (DFW) to explore how AI and machine learning can make ground operations safer, more efficient, and more sustainable.
Acubed led early efforts by Airbus to explore options for developing new eVTOL aircraft, through its work on the Vahana technology demonstrator conducted in tandem with the CityAirbus program at its sister company Airbus Helicopters. Airbus is since committed to developing the four-passenger CityAirbus NextGen aircraft that it aims to start flight testing in 2024.
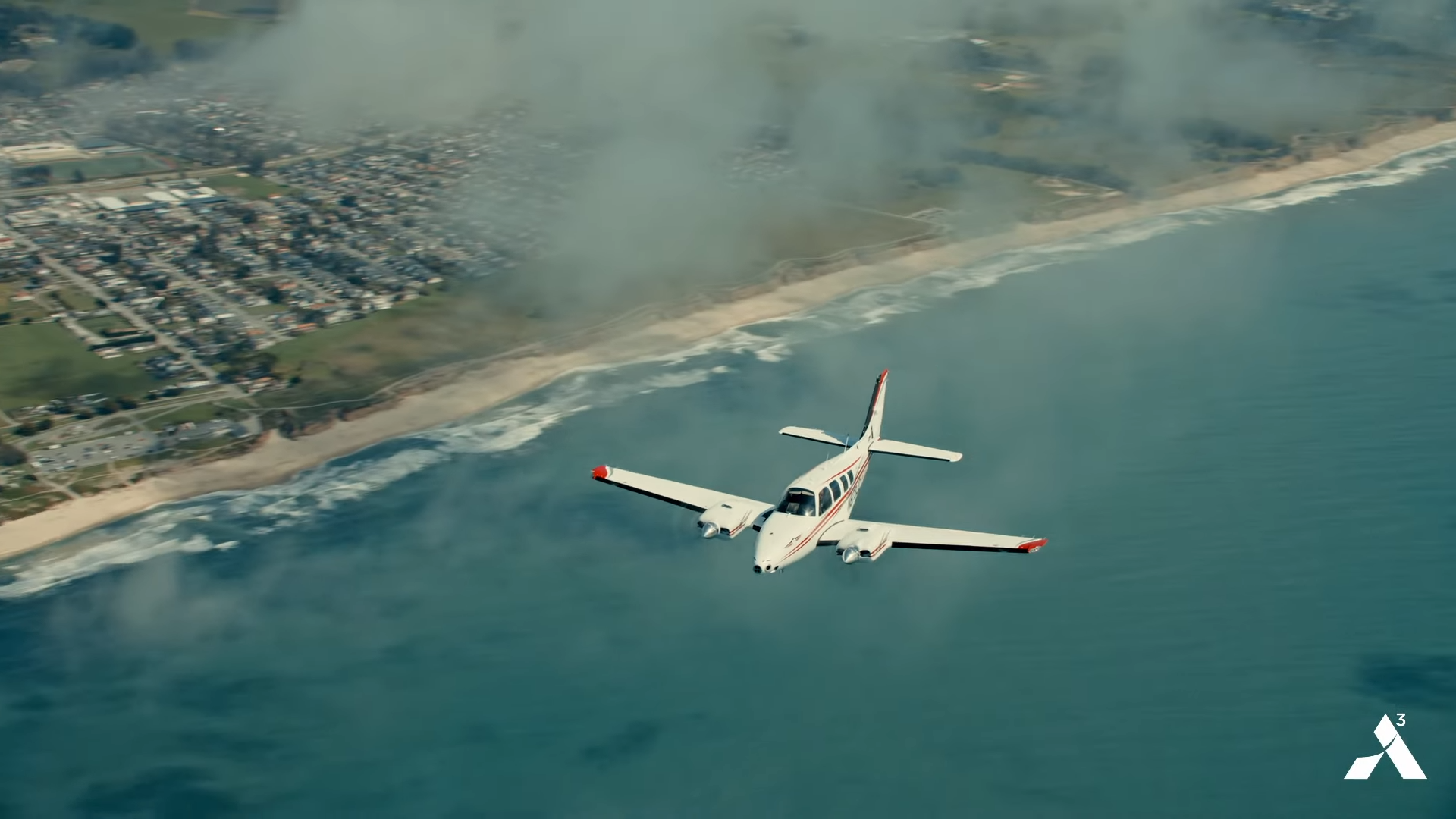